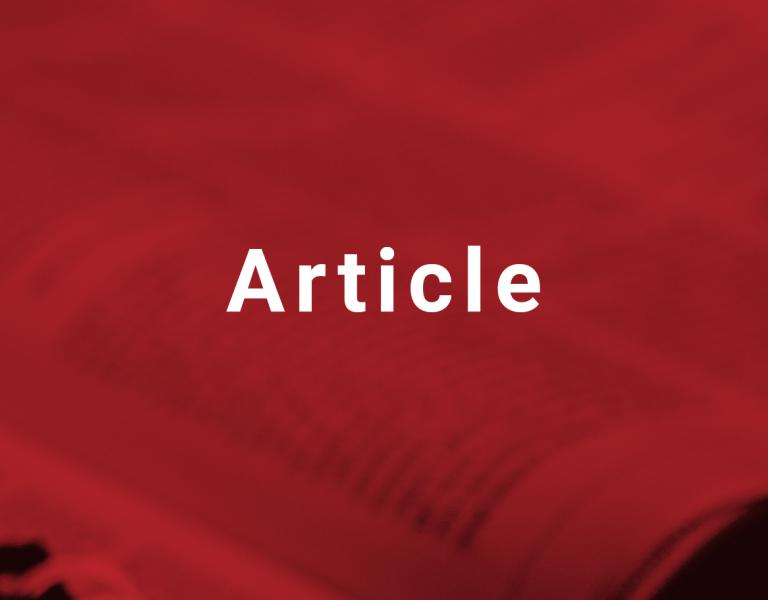
Holistic Life-Cycle Management and Predictive Analytics
Life-Cycle Management (LCM) provides a comprehensive approach for managing system development and sustainment from inception to disposal. With effective modeling and simulation, a Program Manager can be cost effective when implementing, managing, and supporting a system across its lifecycle, including development, production, fielding, sustainment and disposal. Whereas modeling has always been an important part of Life-Cycle Management, unsupervised machine learning and its use for predictive analytics have increased efficiencies and eroded traditional silos. Using predictive analytics and comprehensive decision support modeling, optimization models, predictive maintenance, and more can help program managers better optimize throughout the system lifecycle.
Maintenance from Acquisition to Sustainment
Maintenance is an important part of ensuring a program meets cost and capability targets as it transitions from acquisition into sustainment. Effective life-cycle management includes understanding system failure modes and properly dimensioning supply and maintenance resources. It also ensures the program finds the right mix of cost and capability early in its lifecycle to achieve desired readiness at an affordable cost. The modeling and simulation tools that Life-Cycle Management software provides ensure success.
Real-time Vehicle Level Data in Sustainment
As the program transitions to sustainment, integrating the initial LCM models with actual operational system data is important. Vehicle level data allows for accurate, actionable predictive analytics and decision support modeling to further positively impact cost and capability.
The Life Cycle Model becomes more efficient by accurately predicting potential failures and connecting these predictions to supply chain and man-power optimization models. The end result is increased operational readiness and lower operating costs for the entire system.
Unsupervised Machine Learning Makes True Predictive Analytics Possible
Predictive analytics is made possible by unsupervised machine learning. This emphasizes the current operational impacts on a vehicle in real time with no need to supervise or modify the model and allows more complex processing. It also identifies previously unknown patterns in the data.
This unsupervised form of Machine learning also helps modeling keep up with mobile systems and platforms. Mobile platforms provide the most complex environment for predictive maintenance. Unsupervised machine learning enables regime analysis to provide context and identify the root causes. It also provides real-time insight into performance, creating dynamic changes across engineering, maintenance, supply chain, man-power and even future acquisitions or modifications. In this way, unsupervised machine learning reduces the model obsolescence found in supervised models defined by historical performance.
The Future of Mission Readiness
Mission success will be dramatically increased by leveraging maintenance personnel’s ability to employ proactive maintenance techniques based on real time data analytics. Collecting onboard data sets, normalizing this data, and moving it into a cloud enabled environment is the key to accomplishing the future requirements for sophisticated vehicle fleets in both the commercial and defense sectors. Future mission readiness will be defined not only by how fast data can be removed from a vehicle, but more importantly, how quick it can be delivered to the appropriate personnel to take action.
As a result, maintenance predicted with machine learning can proactively be deployed based on current operations, supply chains can be primed based on real time analytics and training timelines can be reduced through augmented intelligence capabilities. All of the aspects of the system lifecycle and use can be optimized through additional predictive analytics capabilities when combined with a strong Life-cycle management foundation.